Diffusion and Cognition
Diffusion models are transforming AI—but they are also offering a radical new lens on human consciousness and reality itself. By mimicking how we perceive and create, these systems challenge our fundamental understanding of intelligence and blur the line between the natural and synthetic
Introduction
Generative AI models, including diffusion models, are revolutionizing the field of artificial intelligence (AI). These models provide fascinating new insights into intelligence and the nature of reality.
They operate in ways that resemble how humans learn and form memories, potentially bridging the gap between artificial and biological cognition. Their ability to generate highly realistic simulations blurs the line between real and artificial, raising profound questions about perception and consciousness.
Researchers are applying these models to better understand the brain and cognitive processes, which could lead to breakthroughs in neuroscience and treating neurological disorders. The economic potential is immense, with generative AI projected to add trillions in annual value globally.
How Diffusion Models Work
Diffusion models use a unique approach to generate highly realistic outputs:
- Corrupting data with noise in a step-by-step process
- Learning to reverse this corruption to recreate the original data
- Capturing the underlying patterns and structure in the training data
- Generating new data that closely resembles the training examples
This enables diffusion models to generate amazingly lifelike images, audio, and text - often superior to other techniques. The models can extract the essential elements from input data to produce new creative combinations.
Connections to Human Cognition
The way diffusion models operate has intriguing parallels to human cognition. Iterative refinement, and in particular, moving from fuzzy perceptions to clear concepts, is similar to how we learn through experience over time. We analogize the way we use associative memory, which is essentially storing and retrieving information based on associations between concepts. This mirrors how human memory works.
Dimensionality reduction is the key to modeling the world through inference. Algorithms discard irrelevant noise to focus on core features and patterns, enabling abstraction and generalization. This is also central to the diffusion and generation process.
These similarities suggest diffusion models may be replicating aspects of biological intelligence. Studying them could provide a window into our own cognition.
Implications for Reality
As generative AI becomes more sophisticated, it challenges our notions of what is "real". Simulations may become indistinguishable from physical reality. Artificial experiences could feel as authentic as "real" ones. The ability to emulate consciousness raises questions about machine sentience.
While we are still in the early stages, these models force us to reexamine the fundamental nature of reality and subjective experience. What then does it mean if an AI can perfectly replicate any aspect of the world or the mind?
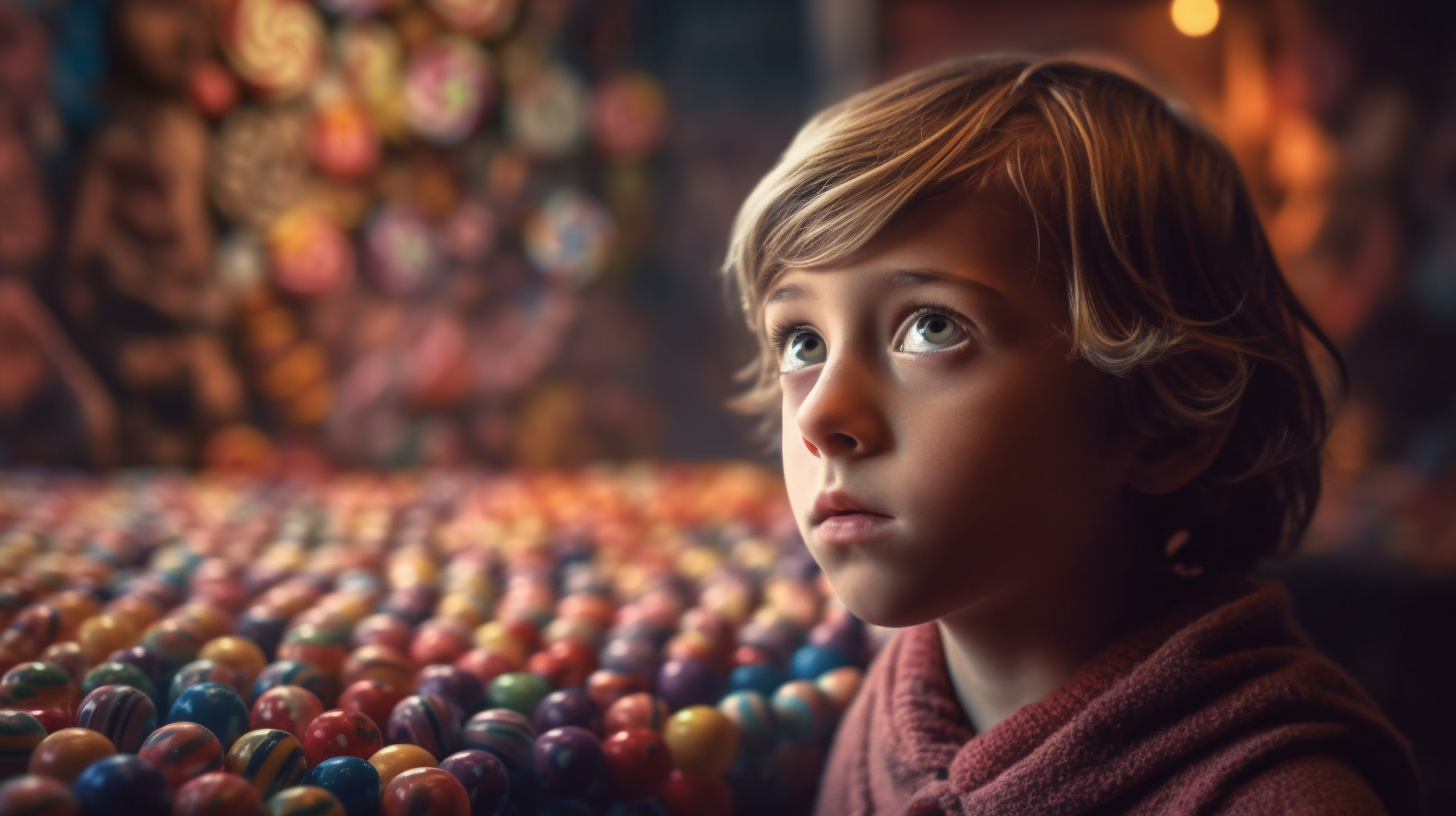
Challenges and Future Directions
Current limitations of diffusion models include:
- High computational requirements for training
- Difficulty with certain data types like text
- Lack of robust evaluation metrics compared to human judgment
However, researchers are making rapid progress in improving the efficiency, flexibility, and assessment of these models. As they mature, diffusion models are poised to make a transformative impact across industries - accelerating scientific discovery, enhancing creative workflows, and unlocking new economic opportunities.
Broader Implications
Ultimately, generative AI offers a powerful lens for understanding intelligence and reality. As we push the boundaries of what is possible with these systems, we will be forced to confront deep questions about the nature of the mind, perception, and consciousness.
Society will also need to grapple with the profound effects this technology may have on the economy, creativity, and truth itself. Establishing best practices for the responsible development and deployment of generative AI will be critical.
We are entering uncharted territory, where the line between artificial and real begins to fade. By thoughtfully examining the implications and continuing to drive the technology forward, we can harness the vast potential of generative AI to better understand ourselves and shape a more prosperous future.